Hi, Here is
Weizhi Tang
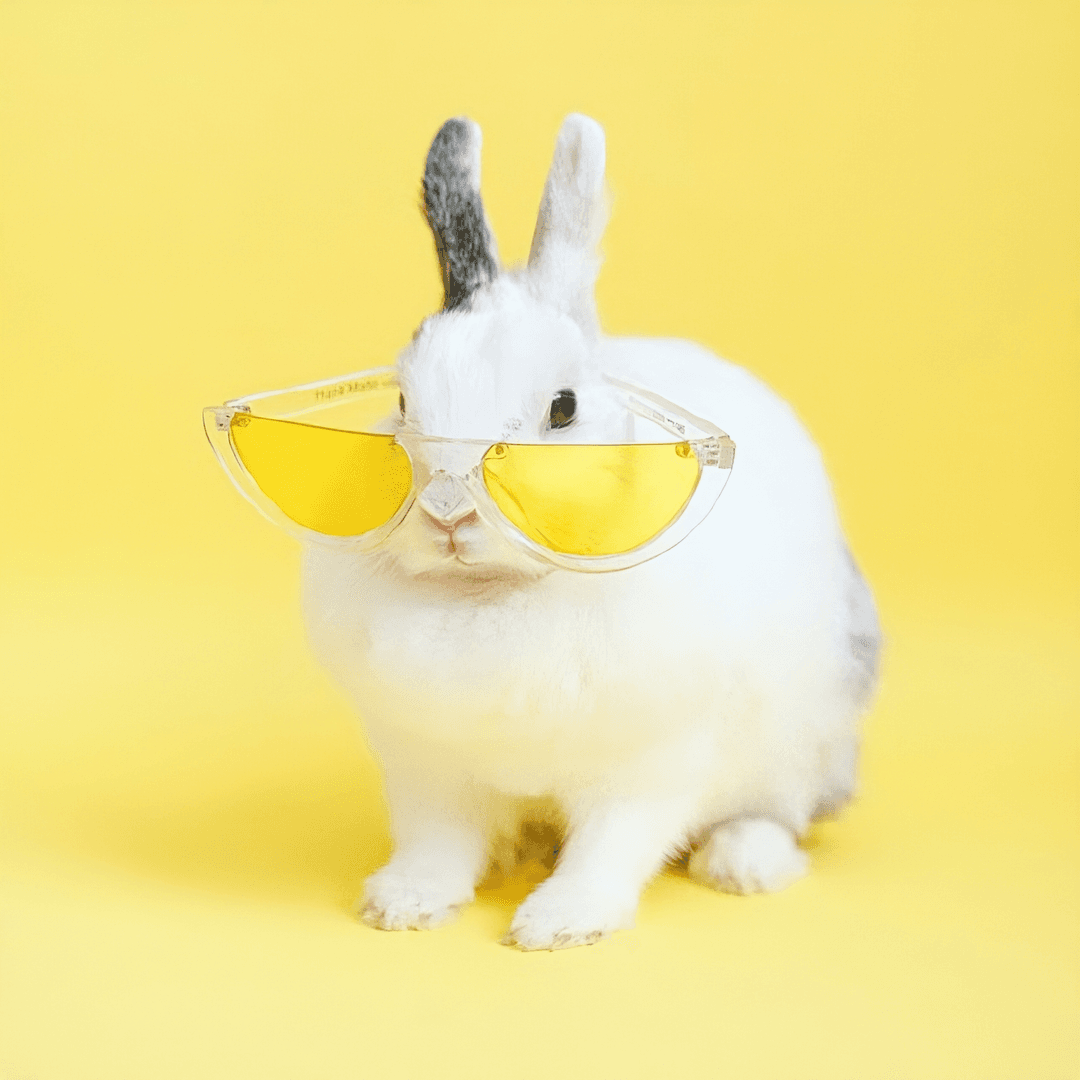
A Researcher in Neuro-symbolic AI and Cognitive Science; A Developer in Frontend, iOS, Backend, and Cloud.
BLOGS π¨π»βπ»
Test Blog 1
Oct, 2024
Lorem ipsum dolor sit amet, consectetur adipiscing elit. Praesent nec molestie nunc, et porttitor risus. Cras rutrum tortor id viverra sagittis. Nam rhoncus tincidunt dui non efficitur. Nullam at erat nunc. Morbi mollis cursus ligula, in vestibulum justo iaculis sed. Aliquam interdum erat tortor, ut egestas nisl tristique vel. Duis lectus enim, lobortis id dictum sed, tristique porta felis. Donec elementum, turpis sed congue suscipit, neque lorem gravida diam, quis consequat augue odio eu sem.
PAPERS π
LTLBench: Towards Benchmarks for Evaluating Temporal Logic Reasoning in Large Language Models
Temporal reasoning (TR) is a critical component of artificial intelligence, encompassing understanding and processing temporal information and relationships between events. To discover and study the TR ability in Large Language Models (LLMs), various datasets have been constructed in different ways for evaluating various aspects of TR ability. Our work proposes a novel approach to design and develop a pipeline for constructing datasets to evaluate the TR ability of LLMs by leveraging random directed graph generation, LTL formula, and the NuSMV model checker. Based on the pipeline, we have also constructed a dataset as a benchmark, namely LTLBench, consisting of 2,000 TR challenges and evaluated six LLMs with it. Furthermore, we have conducted additional experiments to discover the impact of increasing the number of events and formula operators on the complexity of TR problems and the performance of LLMs. We have demonstrated that although LLMs exhibit some promise in handling TR challenges, they still struggle with complex TR. We expect this work can offer insights into TR ability in LLMs while also providing a valuable tool for future TR evaluations.
- Preprint Date: Sun Jul 07 2024
ToM-LM: Delegating Theory of Mind Reasoning to External Symbolic Executors in Large Language Models
Theory of Mind (ToM) refers to the ability of individuals to attribute mental states to others. While Large Language Models (LLMs) have shown some promise with ToM ability, they still struggle with complex ToM reasoning. Our approach leverages an external symbolic executor, specifically the SMCDEL model checker, and fine-tuning to improve the ToM reasoning ability of LLMs. In our approach, an LLM is first fine-tuned through pairs of natural language and symbolic formulation representation of ToM problems and is then instructed to generate the symbolic formulation with a one-shot in-context example. The generated symbolic formulation is then executed by the SMCDEL model checker to perform transparent and verifiable ToM reasoning and give the final result. We demonstrate that our approach, ToM-LM, shows a significant improvement over all the constructed baselines. Our study proposes a novel view about externalizing a particular component of ToM reasoning, mainly reasoning about beliefs, and suggests generalizing it to other aspects of ToM reasoning.
- Published Date: Tue Sep 10 2024